Heartland Forward knows that regions matter.
Taking an aggregate view of any change to the U.S. economy masks the opportunities and challenges faced by regions both rural and urban, as well as some states. It is tempting to think from a national or international angle about such shifts, but it is largely state-level leaders whose actions can mitigate the impact on individuals, families and communities.
Much remains uncertain about the effects of generative artificial intelligence (AI) and large language models (LLMs). Nevertheless, learning more about how the adoption of AI tools might affect employment and opportunities can only help as heartland states work to identify policies and programs that can best help their communities optimize the transition.
Understanding LLMs — the new tools powered by AI
The idea that AI will seriously impact the labor market has gained traction with the introduction of some powerful new tools in the past year.
LLMs like OpenAI’s GPT-4 can return natural language responses to complex questions through its interactive chatbot, ChatGPT. Drawing on vast swathes of data and previous examples, LLMs can also complete many tasks faster than a human. For example, it can generate a custom letter conveying specified information, or provide highly relevant examples and explanations to questions on a specific topic. This ability to substitute for a worker in generating at least a first draft has rung alarm bells about the potential for LLMs to reduce the need for certain positions while increasing the productivity of others. As interacting with LLMs happens using non-specialist language, adoption of this new technology has happened quickly among a wide variety of users.
Perhaps the way to think about ChatGPT is that it reveals natural human language to be far more simple and structured than we once thought, and that feeding a wealth of electronic content to a probability algorithm can provide useful answers to well-written prompts — without needing understanding.1 This capability alone will affect the need for many white-collar jobs, first at entry level and increasingly moving up the org chart with improvement to our prompt-writing skills, as well as the LLM’s ability to generate useful responses.
The labor market will feel this in several ways. Initially, some of the more-routine data collation jobs will be easily replaced by the superior search and data-description capabilities of LLMs. Losing these entry-level or internship opportunities will make entering certain fields more difficult, as it collapses the lower rungs of the ladder. The ability to directly access information with a query written in English, rather than needing to request it from an analyst with specialized knowledge of the data sources, will give more senior employees quicker access to needed information. In the longer term, job responsibilities will adapt to account for LLMs, just as they have as a result of improved tools available for task automation, search, calendaring, photo editing, data analysis, etc. The widespread interest and adoption of LLMs will further speed along these changes, and states must consider how this will impact their labor forces and employment opportunities. Economists have developed various approaches to try estimating the impact the adoption of AI tools could have on the workforce, and we will explore one of these approaches and its expected implications for heartland states.
Broader access to advanced tools like LLMs and the information and analysis they can make readily available to non-specialist users will also open the doors for entrepreneurs. Experience has shown us that new jobs will emerge in the long term, but there will also be short-term discomfort for people dislocated during the transition. Opportunities for entrepreneurs, firms, and industries in heartland states will be discussed more deeply in future work.
Globalization as an analogous, large-scale disruption
The scale of potential change to the labor market goes beyond anything we have seen to date with technological progress — actually, it is more akin to the impact of globalization on U.S. manufacturing. Looking specifically at the effect of China joining the WTO offers a useful benchmark for AI’s potential impact. The lower barriers to trade clearly affected the availability of goods in the U.S. and their prices. Consumers benefited from lower prices for manufactured goods — see the consumer price index (CPI) for computer and electronic products in the chart below — and lower input costs had positive knock-on effects in U.S. industries further up the supply chain.2
Figure 1: Urban Consumer Price Index vs. Manufacturing Employment: Computer and Electronic Products (1998-2022)
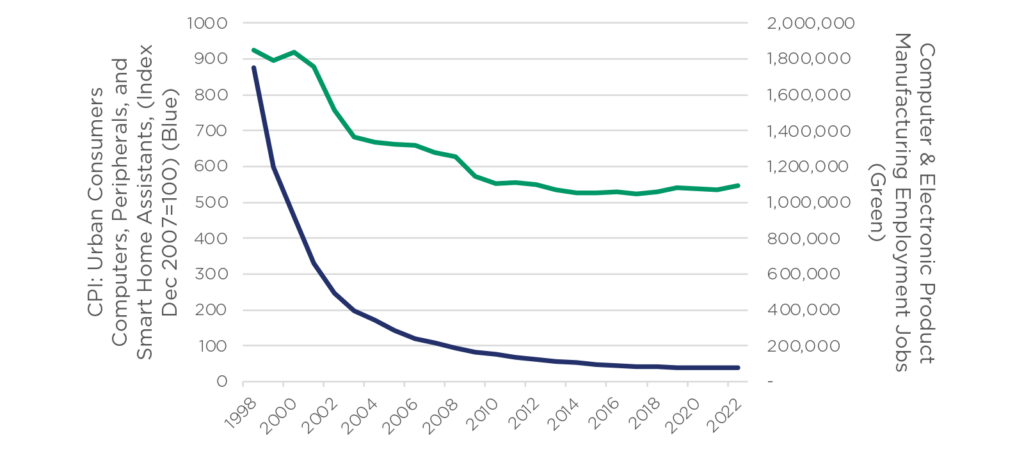
Before China joined the WTO and globalization kicked into a higher gear, many feared the impact of China’s participation on manufacturing jobs in developed countries. The overall welfare of developed countries may have increased with access to cheaper goods and inputs; however, some communities suffered disproportionately from deindustrialization and widespread job losses, as their anchor industries died or relocated production abroad. Cheap labor in other parts of the world created competition that profoundly affected employment in some heartland states.
Globalization also foregrounded the differential impact change can have on the labor force based on skill level. While technological advances and increased productivity were the primary drivers of the decline in manufacturing jobs in the U.S., trade with China is estimated to have contributed to 10% of it.3 As the number of available jobs in certain industries dropped, workers in those industries experienced higher levels of job churn. In general, highly skilled workers transitioned into different industries, while others of lesser skill more typically shifted from employer to employer, competing for the shrinking pool of jobs in the affected industry. Regions with a higher concentration of manufacturing jobs experienced the knock-on effects of unemployment and lower incomes in their communities. Many industries recovered from the shock of globalization, but many communities did not.
Estimating the scale of the potential impact on employment and opportunity
In contrast to how globalization and increased productivity transformed U.S. manufacturing, generative AI will more likely affect higher-skill occupations. Goldman Sachs estimates that 46% of office and administration support positions, as well as 44% of legal jobs, could eventually be performed by AI.4 Based on May 2022 data and taking all legal-service jobs as a pool to provide a rough estimate, that would put about 165,500 legal jobs in heartland states at risk.5 For office and administrative support occupations, this method suggests that 3.385 million additional jobs in heartland states could be affected.
Many heartland states have low concentrations of legal-services jobs compared to the national average — most are between half and three-quarters as concentrated as the U.S. average, with 12 states having location quotients (LQs6) between 0.5 and 0.75, and only two coming in higher than 1 (at 1.03 and 1.05). Regional economies elsewhere in the U.S. are more likely to experience major labor disruptions from AI deployment, as we will explore using more detailed estimates based on specific occupations in future work. Looking beyond the job losses, access to the services currently provided by people in these at-risk jobs will increase and drop in cost. Where AI tools can serve as a substitute for financial analysts, for example, entrepreneurs outside of the core financial-services clusters will have expanded access to high-quality financial analysis to help them grow their businesses. Across the many affected sectors, this shift in availability and affordability could close the support-services gap between rural and urban ventures. The availability of new AI-powered tools will help create new products and services, new firms, and eventually new industries. While we cannot know what these will be, thinking through how heartland states can position themselves to host and benefit from these opportunities is critical for future economic vitality.
ENDNOTES
- Wolfram, S. (2023). What Is ChatGPT Doing… and Why Does It Work?. Stephen Wolfram.
- U.S. Bureau of Labor Statistics, Consumer Price Index for All Urban Consumers: Computers, Peripherals, and Smart Home Assistants in U.S. City Average, retrieved from FRED, Federal Reserve Bank of St. Louis; U.S. Bureau of Labor Statistics, All Employees, Computer and Electronic Product Manufacturing, retrieved from FRED, Federal Reserve Bank of St. Louis
- Autor, D. H., Dorn, D., & Hanson, G. H. (2016). The China shock: Learning from labor-market adjustment to large changes in trade. Annual review of economics, 8, 205-240.
- Hatzius, J., Briggs, J., Pierdomenico, G., & Kodnani, D. (2023). The recent emergence of generative artificial intelligence (AI) raises whether we. Goldman Sachs. https://www.ansa.it/documents/1680080409454_ert.pdf
- U.S. Bureau of Labor Statistics. (2022). Occupational Employment and Wage Statistics. U.S. Bureau of Labor Statistics. https://www.bls.gov/oes/data.htm. Selected states
- A location quotient (LQ) describes in one metric the relative concentration of an economic variable locally as compared to the nation. It can be used to show how much more employment, for example, is supported by a particular industry in a regional economy than the national economy. LQs above 1 mean the industry is more concentrated locally than nationally, LQs under 1 mean that it is less concentrated locally than in the national economy. High LQs can be used to identify a potential cluster and/or exposure to big swings in that particular industry.